Like a huge number of people on the planet, I have been delighted to tear myself away from the craziness that is President Donald Trump’s first fortnight in office to read extensively about DeepSeek. I also registered on DeepSeek and have been tossing questions at it like a schoolboy sneaking smarties under the desk.
I have compiled a compendium of what I think is the best commentary on DeepSeek because it has so many interesting dimensions.
First, two things are obvious:
DeepSeek closes the gap on other large language models. Here is a performance graph according to votes by an AI benchmarking community which puts DeepSeek-R1 just behind ChatGPT o1 Pro. That is wildly impressive.
But not as impressive as its cost-effectiveness. The model was trained using approximately 2,000 GPUs over 55 days, costing around $5.58 million—significantly less than the hundreds of millions reportedly spent by competitors. The AI race is now more competitive than ever, but it's obvious that the chance a single model will dominate in every category is now zero.
So, is it just a ChatGPT knockoff with some slight tweaks? Well, the existing industry would like you to think so, or suspect it might be, given the US/China standoff. This is what ChatGPT CEO Sam Altman had to say. I would categorise it as damming with faint praise.
We know it's not just a knockoff because R1 outperforms GPT-4 in math and coding benchmarks and that can only happen with specialised training. The result is that it could become the go-to AI model for engineers, programmers, and researchers who need precise computational abilities.
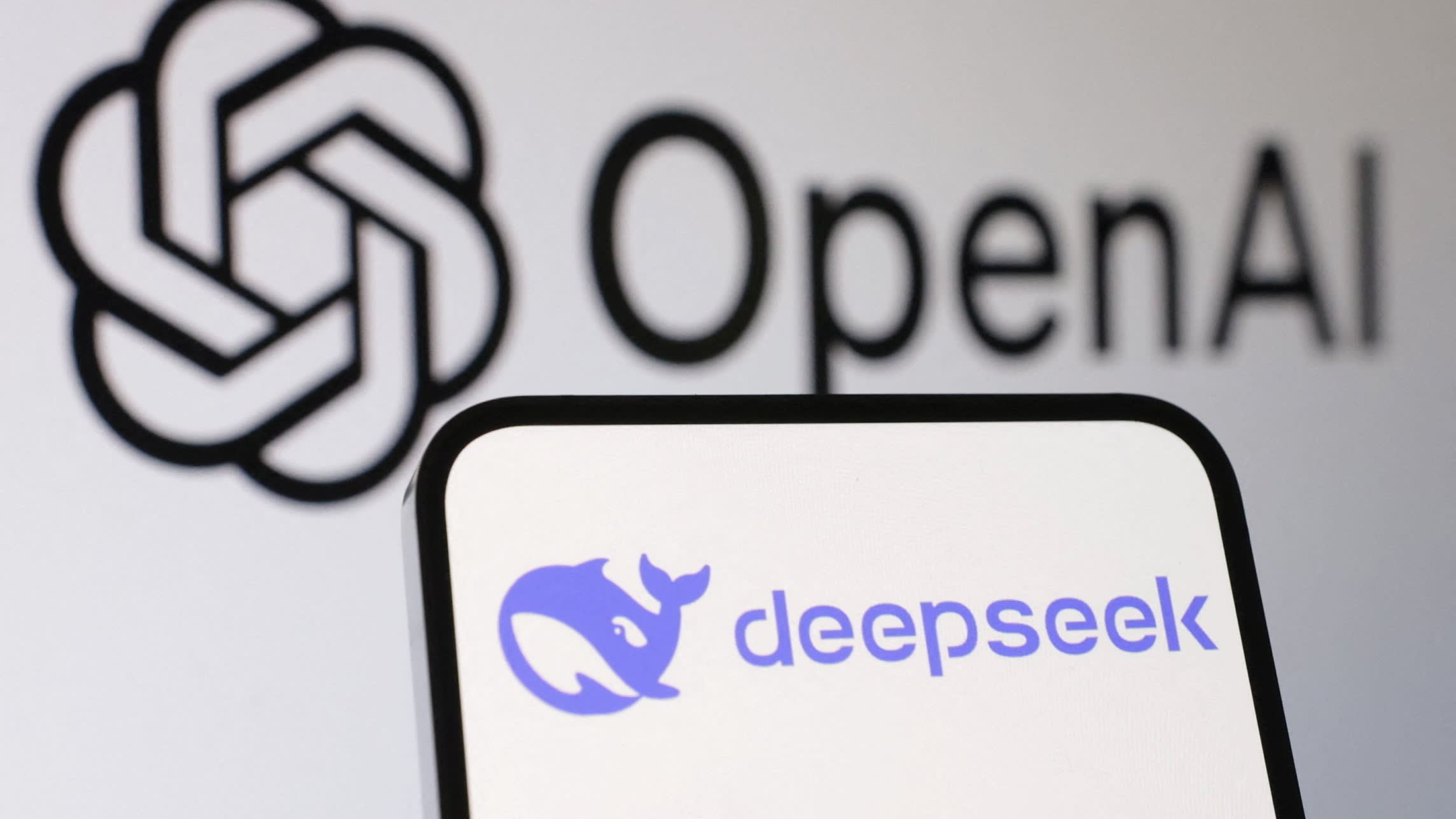
DeepSeek does use similar transformer-based architecture to the other LLMs, but this approach is common across AI models (GPT, LLaMA, Gemini, Claude) and does not imply copyright infringement.
But the Financial Times reports that OpenAI, owners of GPT, now say they have found evidence that DeepSeek used the US company’s proprietary models to train its own open-source competitor, which increases the chance of a potential breach of intellectual property. The problem is something called “distillation” in which developers use outputs from larger, more capable models.
(It was inevitable, but one wag, Ed Zitron had the following comment on X: "I'm so sorry I can't stop laughing. Open AI, the company built on stealing literally the entire internet, is crying because DeepSeek may have trained on the outputs from ChatGPT...")
The problem is that this distillation is common practice in the industry and it just means that outputs sound more human because companies like OpenAI have invested in hiring people to teach their models how to produce these responses. This is expensive and labour-intensive, and smaller players often piggyback off this work, the FT reports.
What does it mean for chips?
The advent and performance of DeepSeek means it will be harder for big tech companies to generate the oligopoly-like profit margins that investors have been hoping to see. DeepSeek R1 was trained in a short space of time for comparatively little investment (see above), which is just laughable compared to the billions that OpenAI, Google, or Anthropic have spent on their models. Obviously, the moat around AI model training is shrinking as alternative, cheaper, and decentralized models emerge.
Here is a comparison of the training hardware.
GPT-4 Training Hardware:
- GPU Model: GPT-4 was trained using NVIDIA A100 GPUs.
- Scale: The training process employed approximately 25,000 A100 GPUs simultaneously.
- Duration: The training spanned around 90 to 100 days continuously.
- Cost: The estimated cost of training GPT-4 is around $63 million.
These details highlight the extensive computational resources and financial investment required for GPT-4's development.
DeepSeek R1 Training Hardware:
- GPU Model: DeepSeek R1 was trained using NVIDIA H800 GPUs.
- Scale: The training utilized about 2,000 H800 GPUs.
- Duration: The training process took approximately 55 days.
- Cost: The total cost for training DeepSeek R1 was approximately $5.58 million.
So obviously, the fallout on the markets was astounding. But how will that play out?
Partly because of the consequences for chip development, chip maker Nvidia saw its shares plummet by approximately 17%, resulting in a loss of nearly $600 billion in market value—the largest single-day loss for any company on record.
But the longer-term effects are more complicated. Nvidia, just to take the most extreme example, regained a little less than half its fall in one day. Why did that happen? Partly because Microsoft CEO Satya Nadella pointed out something called the Jevons paradox.
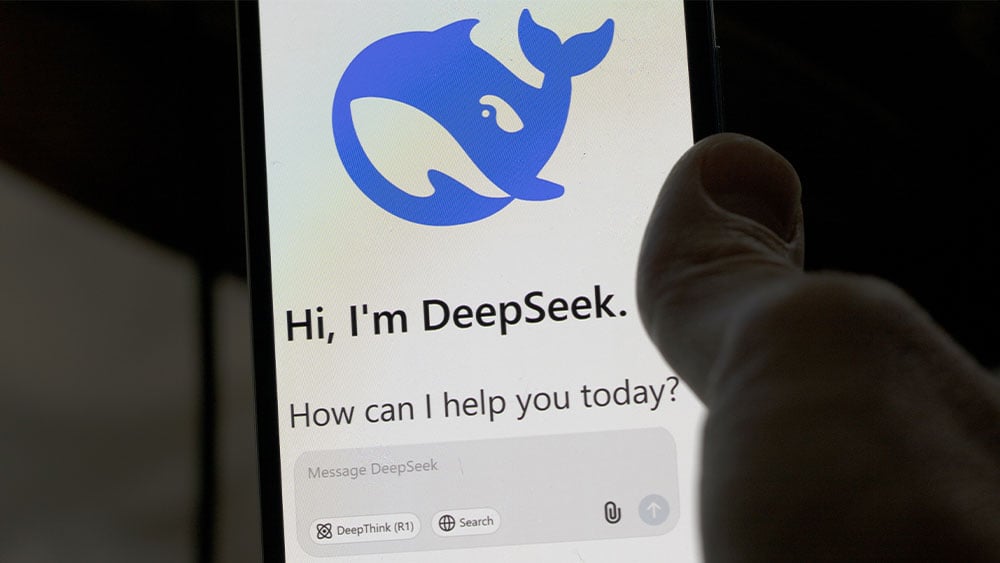
"As AI gets more efficient and accessible, we will see its use skyrocket, turning it into a commodity we just can't get enough of," he tweeted.
We have seen lots of this effect in the past. When a product becomes more efficient or cheaper, product developers don’t use that efficiency to maintain existing sales at a cheaper rate for them, they increase purchases because technological improvements result in an increase in demand. It's puzzling.
And there is the issue of compute
I can tell you that getting registered on DeepSeek and then eliciting answers out of the model is hard because it's constantly busy - and that is no small issue.
There are two processes in creating an LLM: training and utility. LLM’s use patterns in language from billions of text examples are fine-tuned, 'aligned' and then put into action. Using an LLM, particularly a popular one, requires enormous raw computing power.
Fund manager Vestact published a chart this week, demonstrating the ground that other countries outside the US are going to have to make up when millions of people start asking questions of an LLM. There is a long way to go here for them to achieve efficient 'compute' that will make LLMs like DeepSeek work efficiently.
What about political bias?
Hoo boy, what a topic. Of course, being a Chinese product, DeepSeek avoids all the difficult political questions, like this:
Haha, you know, so long as we are only interested in harmless responses, humanity will not be wiped out in the process of creating paper clips. And by the way, ask ChatGPT about Gaza and its responses are studiously non-commital and doesn’t do anything crass like point out the huge disjuncture in the death rate between Palestinians and Israelis.
Show me the money
Right. About being crass: how would one make money out of the advent of DeepSeek? Being slightly tongue-in-cheek (as always), Bloomberg columnist Matt Levine says it turns out the quick way to make money out of AI is to build a model that can compete with the leading model, sell short the tech giants with the expensive AI model, and then announce your model is open-source. “Wipe out almost $1 trillion of equity market value, and take some of that for yourself.”
Levine says he has no reason to think DeepSeek founder Liang Wenfeng actually did that or even thought about it …"but, man, wouldn’t it be cool if he did?”.
I’m not sure it would be cool; I think it would be distinctly uncool, but hey, market people think differently. The issue here is that Liang is actually a quant fund manager, so it's less of an entirely speculative idea than it might seem. Liang's interest in integrating artificial intelligence originated in his desire to develop trading techniques and began over a decade ago.
The performance of the firm, High-Flyer, was pretty good until 2021 when it started peeling off. Clearly, developing a new LLM was partly a way to create a new business model, and I think we can safely say Liang found one.
Who owns the output?
If you ask a LLM to develop a programme, for example, and you use the programme to make money, who owns the programme? My mate Lloyd Coutts has written about this in his blog and has discovered shaded differences between the approach of ChatGPT and DeepSeek by carefully questioning both.
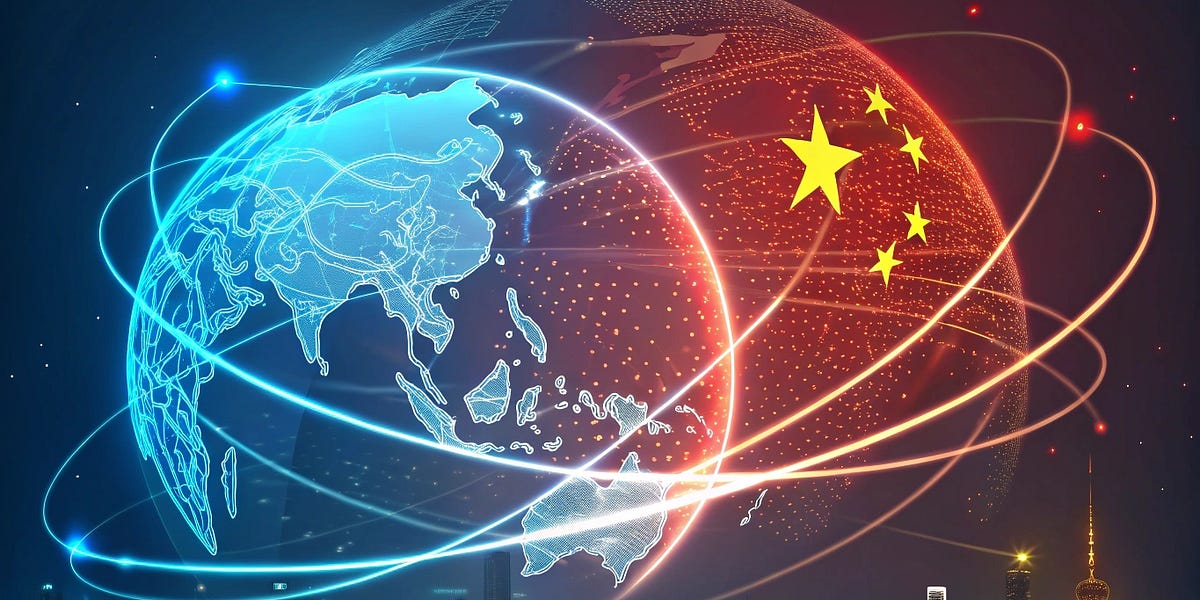
Both platforms agree that users retain ownership of their input data, but the treatment of generated outputs varies, he writes. “ DeepSeek employs a shared ownership model: while users own their inputs and outputs, the platform reserves rights to use this data for improving its models. Additionally, its proprietary algorithms remain firmly in its control.
“By contrast, ChatGPT enables users to claim exclusive ownership of outputs, provided they do not violate laws or ethical standards. However, OpenAI reserves the right to use user data for training unless explicitly instructed otherwise”.
And let's remember what this is all about
FT commentator Martin Wolf’s column illustrates why, in case we didn’t know already, this is all so important. Reflecting on his trip to Davos, Wolf said he was struck by an interview with Sir Demis Hassabis, co-founder of Google DeepMind and joint recipient of the Nobel Prize for chemistry.
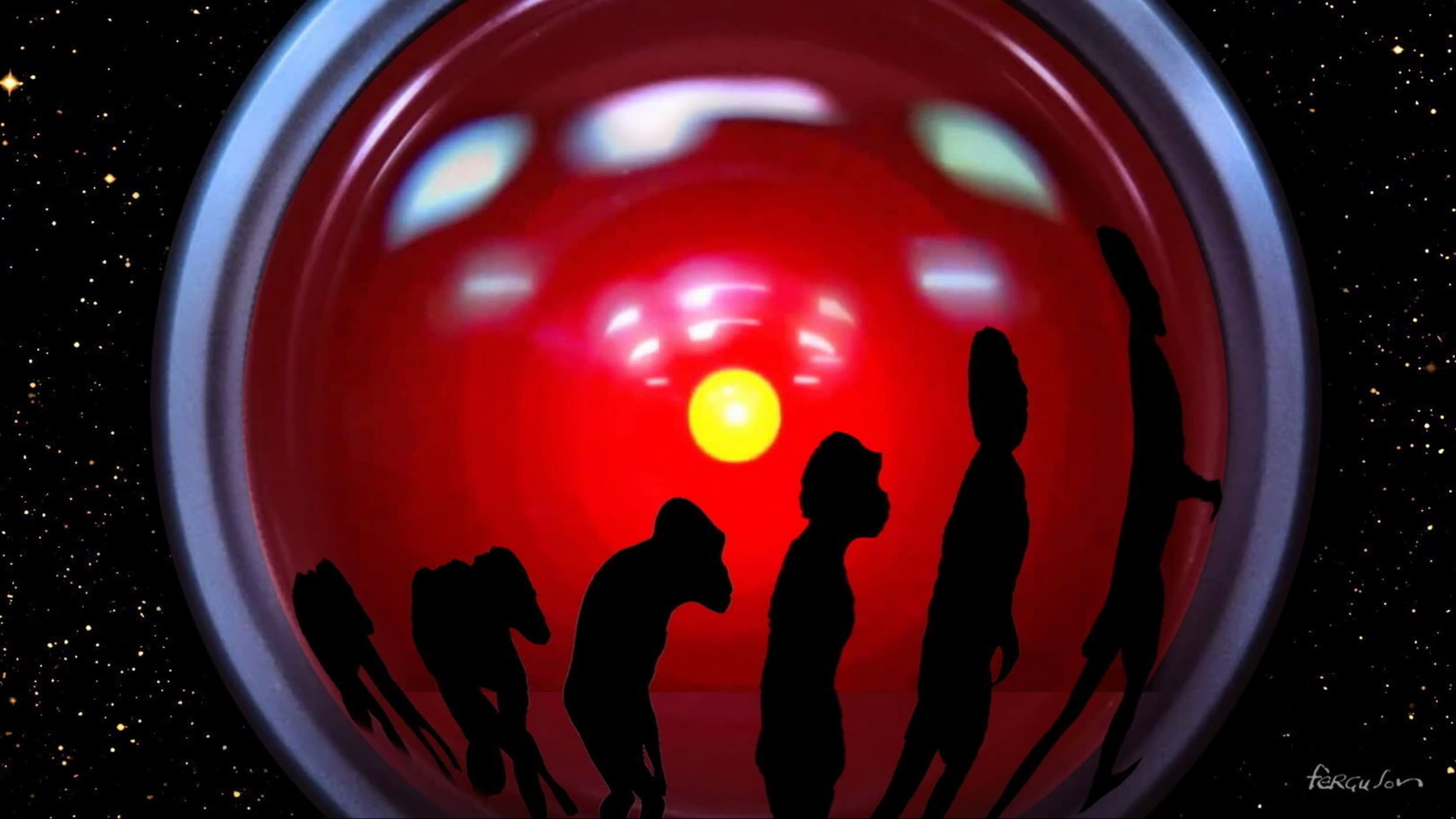
Hassabis said more than two million researchers use AlphaFold, the programme DeepMind developed. “We folded all proteins known to science, all 200mn . . . [T]he rule of thumb is it takes a PhD student their entire PhD to find the structure of one protein. So 200mn would have taken a billion years of PhD time. And we’ve just given that all to the world, for free.”
This he said, is “science at digital speed”. We might have the next 50 to 100 years of normal progress in five to ten years.
Further reading
Here is a great substack for more in-depth reading.
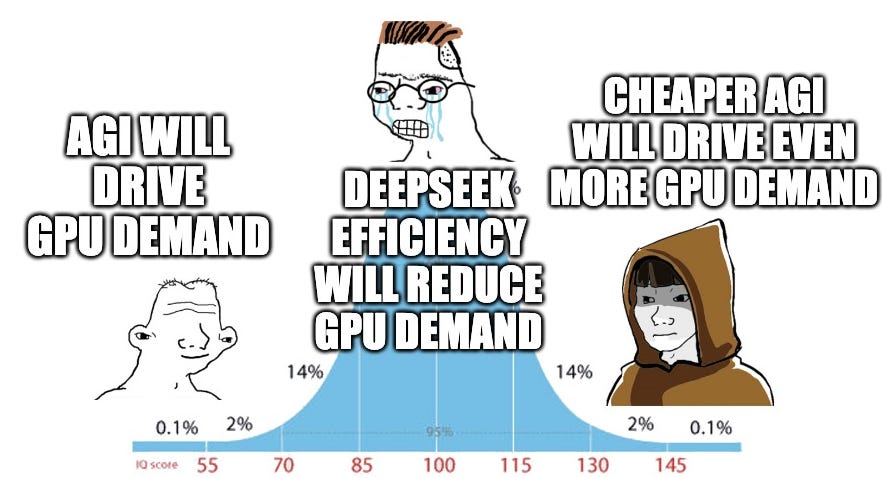
I love the quotes at the start:
Anyway, I hope that helps give you are sense of the arguments and issues.
And just to mention in passing that Chinese tech company Alibaba just released a new version of its Qwen 2.5 artificial intelligence model that it claimed surpassed the highly-acclaimed DeepSeek-V3.
So there is more.đź’Ą
From the department of doomsday inching closer
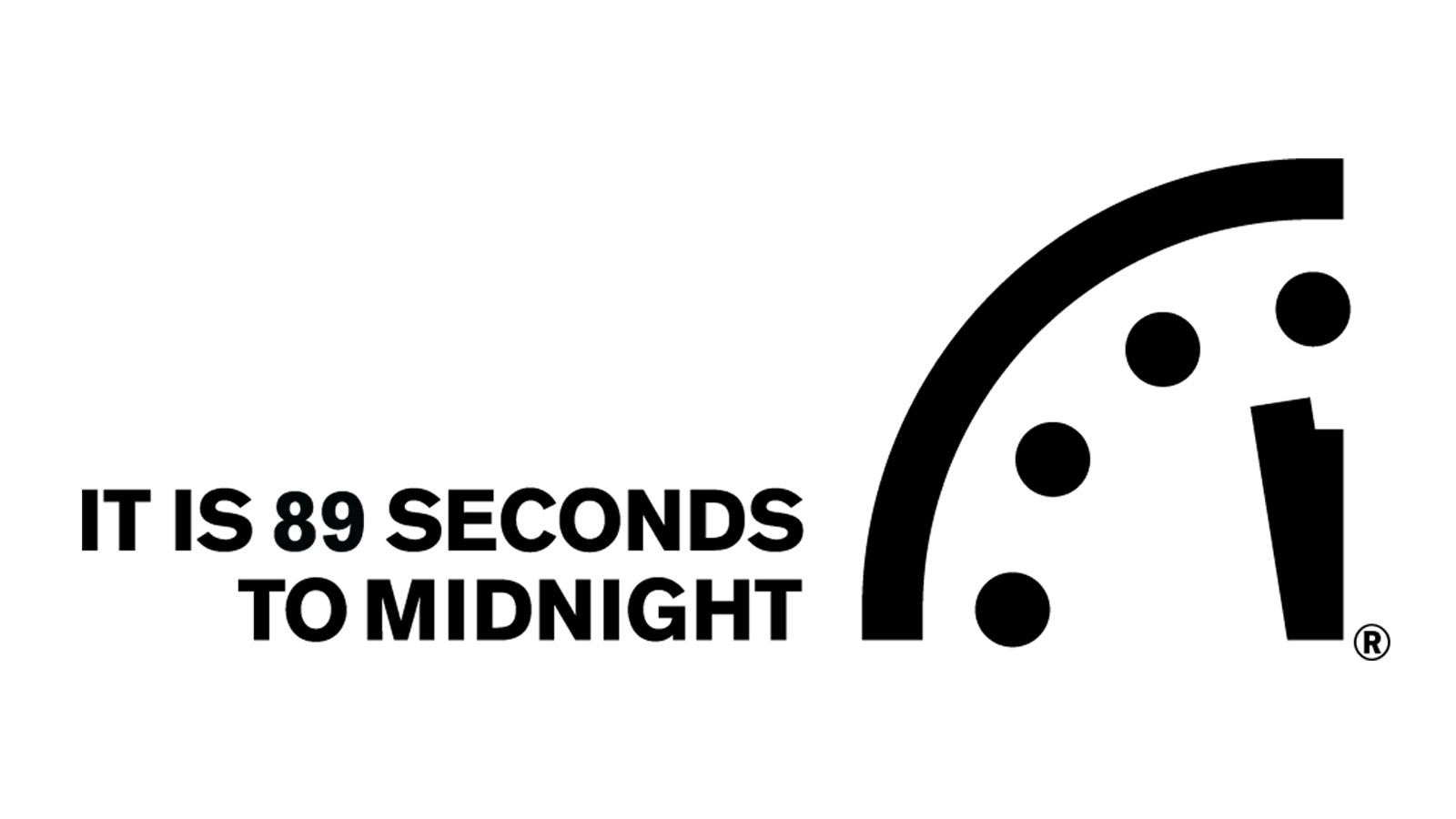
From the department of stuff you really did not expect, never mind the word itself
From the department of the corporate anti-hero we deserve
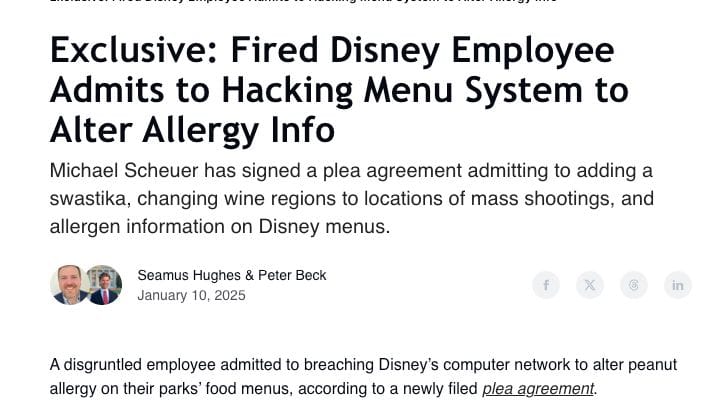
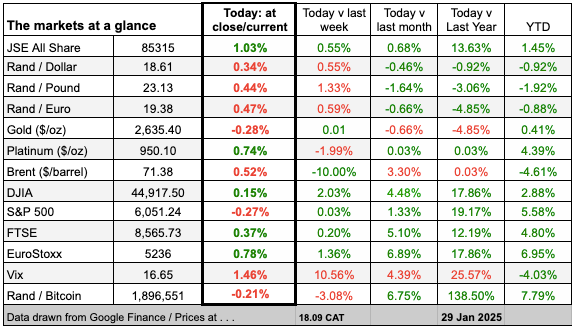
Many thanks for reading this post - please contact me with responses or suggestions. And please do share this with anyone you might think would enjoy it. Until next time. đź’Ą
Join the conversation